Machine Learning & Intelligent Control Lab.
Principal Investigator
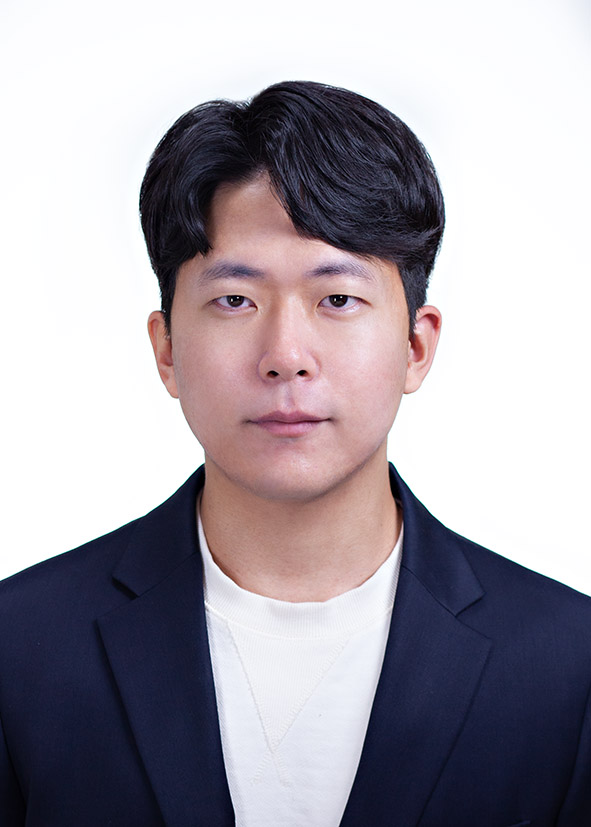
Seungyul Han
Assistant Professor
AI Graduate School (AIGS) & Department of Electrical Engineering (EE)
Ulsan National Institute of Science and Technology (UNIST)
50 Unist-gil, Ulsan, 44919, South Korea
Biography
Seungyul Han is an assistant professor in the Artificial Intelligence Graduate School (AIGS) and in the department of Electrical Engineering (EE) at the Ulsan National Institute of Science and Technology (UNIST). He received B.S. (Double major in Mathematical Science) and M.S. degrees in Electrical Engineering from Korea Advanced Institute of Science and Technology (KAIST), Daejeon, South Korea in 2013 and 2016, respectively. He also received his Ph.D. degree in Electrical Engineering from KAIST. Prior to joining UNIST, he was an postdoctoral researcher at Institute of Information Electronics in KAIST, and he is now an assistant professor in UNIST. His main research interests include reinforcement learning, machine learning, deep learning, multi-agent systems, signal processing, and intelligent control systems.
Research Areas
The research of our group focuses on the development of breakthrough machine learning (ML) algorithms, theoretical improvements based on mathematics, and real-world ML applications for industrial automation. In order to conduct influential research in the core areas of artificial intelligence (AI), we mainly consider the following research topics:
- Reinforcement Learning
- Offline Reinforcement Learning
- Domain Adaptation/Imitation Learning
- Multi-Agent Reinforcement Learning
- Meta Reinforcement Learning
- Robust/Safe Learning
- Statistical Learning
- Intelligent Control systems
- Signal Processing
Offline Reinforcement Learning
- Online-RL requires interaction with the environment, but these interactions are often costly or difficult to perform.
- Offline-RL learns policy by utilizing only the experience it gathers without additional interaction with the environment.
- There are still some challenges, such as distribution shifts and out-of-distribution problem.
Imitation Learning
Imitation learning is a branch of research aiming to apply reinforcement learning to real-life scenarios, focusing on learning policies that mimic the actions of experts. Prominent methods include Behavior Cloning, which learns policies by imitating demonstrations of experts using supervised learning techniques, and Inverse Reinforcement Learning, which estimates reward functions for learning. Recently, there has been progress in research on Domain Adaptation and Cross-Domain studies, enabling imitation of actions from experts in different domains, and a significant amount of research is being conducted to learn algorithms applicable to general situations.
Multi-Agent Reinforcement Learning
In multi-agent reinforcement learning, multiple agents aim to learn policies that would maximize the expected return from a shared environment. Coordination among the agents is essential for achieving this goal as the agents effect themselves as learning progresses. As MARL presents unique challenges compared to single-agent reinforcement learning, including non-stationarity, partial observability, and scalability, the focus of the research lies on maximizing the usage of limited information.
Meta Reinforcement Learning
Even if we humans face a new situation we have not experienced, we quickly grasp the situation and adapt to the situation and take appropriate actions. In this process, we recall and reuse numerous previously learned experiences. Since most reinforcement learning agents learn a policy that maximizes rewards in a single task environment, they only act appropriately for that environment, and when they encounter a new scenario, the policy performance deteriorates. Meta reinforcement learning aims to learn a task-general policy so that reinforcement learning agents can quickly adapt to new situations like humans.
- We are currently looking for highly motivated interns, M.S., Ph.D., and Combined M.S./Ph.D. students in machine learning fields !
(If you are interested, email me your CV and transcript.)
News
[2024/03/01] Isak Park, Sangjun Bae, and Gawon Lee joined our lab !
[2024/01/12] Lectures: LG Innotech LV 3 (advanced AI course), “Deep Reinforcement Learning”.
[2023/12/10] Paper “FoX: Formation-aware exploration in multi-agent reinforcement learning” is accepted to AAAI 2024.
[2023/09/22] Paper “Domain Adaptive Imitation Learning with Visual Observation” is accepted to NeurIPS 2023.
[2023/09/15] Lectures: UNIST AI Innovation Park, “6th AI Novatus Academia, 3/4th AI Novatus Academia 경남.”
[2023/03/01] Sunwoo Lee, Sanghyeon Lee, and Taehyun Ahn joined our lab !
[2023/01/27] Lectures: UNIST AI Innovation Park, “4/5th AI Novatus Academia, 2nd AI Novatus Academia 경남.”
[2022/07/25] Lecture: LG DXI course, “Reinforcement Learning.”
[2022/07/01] Jeongmo Kim, Minung Kim, and Heeseong Eom (intern) joined our lab !
[2022/05/25] Lecture: 한국통신학회(KISC) AI Frontiers Summit (AIFS) Tutorial, “강화학습 및 응용에 대한 최신 연구 동향”
[2022/05/15] Paper “Robust imitation learning against variations in environment dynamics” is accepted to ICML 2022.
[2022/04/01] Project PI: 자율 드론 실용화를 위한 목적지향 강화학습 핵심기술 개발 (IITP 사람중심인공지능 핵심원천기술개발, 22.04 – 26.12)
[2022/03/18] Lecture: UNIST AI Innovation Park, “1st AI Novatus Academia 경남,” Week 3.
[2022/03/01] Yonghyeon Jo and Junghyuk Yum joined our lab !
[2022/01/28] Lecture: UNIST AI Innovation Park, “3rd AI Novatus Academia,” Week 3 & 4.
[2022/01/24] Lecture: 한국통신학회(KICS) 단기강좌 (머신러닝/강화학습의 기초 및 응용 강좌), “강화학습의 기초.”
[2021/11/01] I have joined the Artificial Intelligence Graduate School and the Department of Electrical Engineering at Ulsan National Institute of Science and Technology (UNIST) as an assistant professor.
[2021/09/29] Paper “A max-min entropy framework for reinforcement learning” is accepted to NeurIPS 2021.
[2021/05/08] Paper “Diversity actor-critic: Sample-aware entropy regularization for sample-efficient exploration” is accepted to ICML 2021.